Tutorial 13 | 7th Jan | 9:30AM-12:30PM (IST)
Neuromorphic Systems: From Event-driven sensors to In-Memory Computing Circuits
Speaker: Prof. Arindam Basu, University of Hong Kong
About Speaker:
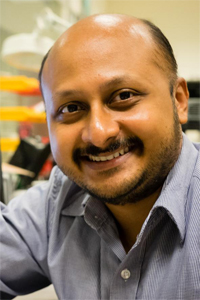
Prof. Arindam Basu received the B.Tech and M.Tech degrees in Electronics and Electrical Communication Engineering from the Indian Institute of Technology, Kharagpur in 2005, the M.S. degree in Mathematics and PhD. degree in Electrical Engineering from the Georgia Institute of Technology, Atlanta in 2009 and 2010 respectively. Dr. Basu received the Prime Minister of India Gold Medal in 2005 from I.I.T Kharagpur. He is currently a Professor in City University of Hong Kong in the Department of Electrical Engineering and was a tenured Associate Professor at Nanyang Technological University before this.
He is currently an Associate Editor of IEEE Sensors journal, Frontiers in Neuroscience, IOP Neuromorphic Computing and Engineering, and IEEE Transactions on Biomedical Circuits and Systems. He has served as IEEE CAS Distinguished Lecturer for 2016-17 period. Dr. Basu received the best student paper award at Ultrasonics symposium, 2006, best live demonstration at ISCAS 2010 and a finalist position in the best student paper contest at ISCAS 2008. He was awarded MIT Technology Review's TR35 Asia Pacific award in 2012 and inducted into Georgia Tech Alumni Association's 40 under 40 class of 2022. He is a technical committee member of the IEEE CAS societies of Biomedical Circuits and Systems, Neural Systems and Applications (Chair) and Sensory Systems.
Tutorial Abstract:
Neuromorphic spiking sensors are inspired by the functionality of their biological counterparts (e.g. retina to inspire vision sensors, cochlea to inspire auditory sensors, mechanoreceptor to inspire touch sensors, etc.) and provide change detection mechanisms at the sensor to directly produce sparse outputs. The asynchronous outputs of these event-driven sensors can enable always-on sensing at possible low-latency system-level response time compared to conventional sampled sensors for Internet of Things (IoT) and Brain-Machine Interface (BMI) applications. Recent developments in deep networks, spiking networks, and in-memory computing have led to very low-power neuromorphic systems that combine these sensors and networks for these application domains. In-memory computing can help to reduce power from off-chip memory access and can additionally, support different learning algorithms useful for adaptive neuromorphic systems. Also, these methods reduce the energy required to implement matrix multiplies, nonlinearities, and other signal-processing operations over digital systems.
This tutorial will describe the advances in the design of neuromorphic sensors, bio-inspired network architectures and algorithms, and hardware implementations that can also be applied to the spiking sensor output. We will present examples of the use of neuromorphic systems in low-latency low-power application domains.