Plenary Sessions
Plenary Session 1: A.I VLSI
Accelerating Deep Learning with Tensor Processing Units
Abstract :
Google’s Tensor Processing Unit (TPU), first deployed in 2015, provides services today for more than one billion people and provides more than an order of magnitude improvement in performance and performance/W compared to contemporary platforms. Inspired by the success of the first TPU for neural network inference, Google developed multiple generations of machine learning supercomputers for neural network training that allow near linear scaling of ML workloads running on TPUv2 and TPUv3 processors. TPUs extend research frontiers and benefit a growing number of Google services.
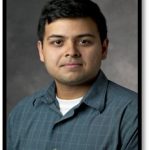
Nishant Patil, Google
Speaker Bio :
Nishant Patil leads system architecture definition, co-design, and performance optimization for Google TPUs informing the strategic direction for Accelerators and Machine Learning Platforms at Google. He has an MS and PhD in Electrical Engineering from Stanford University and a BS in Electrical and Computer Engineering from Carnegie Mellon University.
Machine Learning on Social Network Platforms
Abstract :
Social networks have been deeply woven into our everyday life. These Internet platforms host a plethora of real-time services to keep people connected, provide customized information to users, and preserve information transparency. Underneath their infrastructure, the adoption of Machine Learning (ML) techniques is rapidly becoming omnipresent in both datacenters and end users’ devices, steering a rich feature set to enhance the effectiveness of users’ communication and to improve the quality of online experiences. Meanwhile, to achieve these objectives, ML can consume enormous computing resources and require meticulous resource design, provisioning, and management. In this talk, I will share our vision on the computation technologies and acceleration strategy at Facebook’s infrastructure, in particular, the state-of-the-art of our machine learning approaches on production-scale DNN-based personalized recommendation models for content ranking. Moreover, I will discuss the computing and privacy challenges lying ahead from the perspective of a social network service provider.
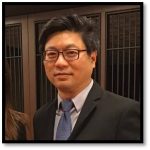
Hsien-Hsin Sean Lee, Facebook
Speaker Bio :
Hsien-Hsin Sean Lee leads the AI Infrastructure Research group at Facebook Boston. Previously, he directed the EDA design flow solutions and oversaw the entire PDK development for IC design customers at Taiwan Semiconductor Manufacturing Co. (TSMC), Taiwan. Prior to TSMC, he was a tenured Associate Professor at the School of Electrical and Computer Engineering, Georgia Tech, an Architecture Manager at Agere Systems and a senior processor architect at Intel. Hsien-Hsin Sean Lee holds a Ph.D. in Computer Science and Engineering from the University of Michigan, Ann Arbor. He was a receipt of the NSF CAREER Award and the Department of Energy Early CAREER Award and has published two book chapters and more than 100 technical articles including 4 Best Paper Awards and one 10-year most influential paper award (ITC). He served as an Associate Editor of IEEE Trans. on Computers, IEEE Trans. on CAD, ACM Trans. on Architecture and Code Optimization, and IEEE MICRO. He also served as the General Chair for IISWC 2010, the Program Co-Chair for MICRO 2016, an Executive Committee Member for IEEE-TCCA, an Industry Advisory Board Member for IEEE Computer Society and a TPC Member for more than 90 international conferences. Hsien-Hsin Sean Lee holds 26 US patents and is a Fellow of the IEEE.
AI Chip Commercialization: From Application to Silicon
Abstract :
We have seen many domain specific architectures (DSAs) for AI computation but only a few of them has gone into mass production. Despite of the quality and yield issue, complete and easy-to-use software stack that maps application onto hardware is a major challenge. This talk presents our work on designing the software stack for our DPU on FPGA. Several different interfaces are designed to allow fast development for developers in different level, while neural network pruning and quantization are embedded. Extendible instruction set is designed to tackle rare neural network architectures. Backend optimization techniques are also introduced. Our work has been used as Xilinx Vitis AI, the unified software stack recently announced by Xilinx.
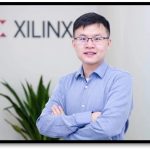
Song Yao, Xilinx
Speaker Bio :
Song Yao is the Senior Director of AI Business in Xilinx. Before joining Xilinx, he was the cofounder and CEO of Deephi Tech, a startup focused on deep learning inference platform and solutions, which was acquired by Xilinx in 2018. Song received BS degree from Department of EE, Tsinghua University in 2015 with honors. He was also a visiting student in Stanford University in 2014. He received many awards including Best Paper Award of FPGA’17 conference, First Prize of Technology Invention from China Computer Federation, and MIT Tech Review Under 35 Innovators Award.
Plenary Session 2: Plenary Fireside Chat: VLSI in India
Moderator
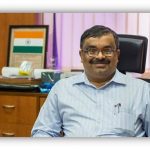
Dr. Partha Pratim Chakraborty
IIT Kharagpur
Panelists
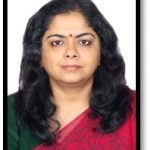
Ms. Anna Roy
Niti Ayog, Govt. of India
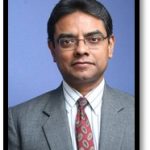
Dr. Pradip Dutta
Synopsys India Country Head
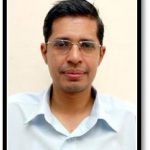
Dr. V Kamakoti
IIT Madras
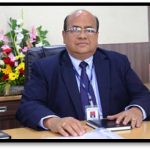
Dr. Hemant Darbari
Director General, CDAC
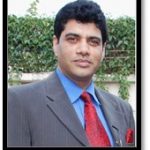
Reapan Tikoo
CEO, Powai Labs
Plenary Session 3: Opportunities and Challenges for Semicon Startups in India
